Content
AP Statistics
AP Statistics is a college-level high-school statistics course offered by the United States of America. This program is run by the College Board. Taking this course is equivalent to taking a one-semester non-calculus-based introductory college statistics course.
Students are taught about different statistical concepts such as probability, random variables, data collection, etc. This will increase a student’s capability in analyzing and drawing a conclusion by using logical and statistical reasoning. This will help students interested in a career in business analytics, data science, meteorology, statisticians, and many more!
If students pass their AP courses, they may receive college credit or an upper-level college placement course. AP courses are generally offered to students in their sophomore, junior and senior years of high school.
Students are taught a variety of new and different concepts related to Statistics. There are 9 units that are covered in AP Statistics. The topics covered in AP statistics are:
Units | Weight % (FOR MULTIPLE CHOICE QUESTIONS) |
Unit Ⅰ: Exploring one-variable data | 15%-23% |
Unit Ⅱ: Exploring two-variable data | 5% -7 % |
Unit Ⅲ: Collecting data | 12%-15% |
Unit Ⅳ: Probability, random variables, and probability distributions. | 10%-12% |
Unit Ⅴ: Sampling distributions | 7%-12% |
Unit Ⅵ: Inference for categorical data: Proportions | 12%-15% |
Unit Ⅶ: Inference for Quantitative data: Means | 10%-18% |
Unit Ⅷ: Inference for categorical data: Chi-square | 2%-5% |
Unit Ⅸ: Inference for quantitative data: Slopes | 2%-5% |
Source: College Board
Looking for last minute help for your AP Statistics exam in May? Find an expert 1-on-1 online AP Statistics tutor from Wiingy and give your exam prep a boost!
AP Statistics exam
AP Statistics exam will test students on their understanding of the mathematical units covered in the course, including their ability to use statistical concepts and reasoning to calculate the probability of an outcome.
The AP Statistics exam is 3 hours long and is divided into 2 sections. Section 1 will test the student’s understanding of concepts from all 9 units. Section 1 consists of 40 MCQ-type questions. Section 2 will test students’ ability to communicate justification using data, definition, and statistical inference. Section 2 consists of 6 free-response questions. Both sections carry equal score weightage.
Students are allowed to carry a calculator with statistical capabilities to the exam.
Some important topics in AP Statistics
Here is a list of some of the important topics in AP Statistics:
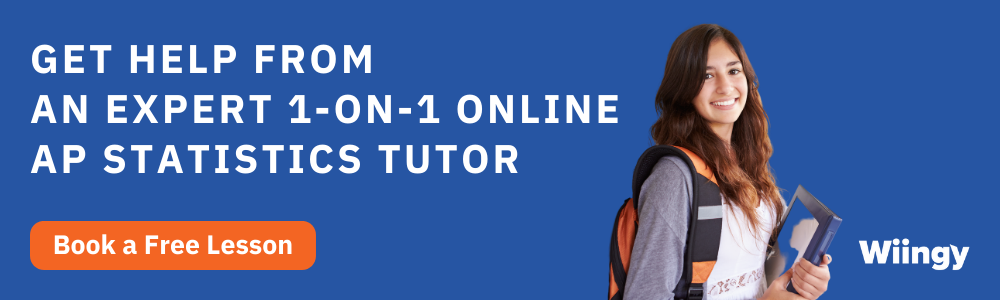
Written by by
Prerit Jain