Content
Geometric Distribution, Definition and Solved Examples
The geometric distribution is a type of discrete probability distribution that represents the probability of the number of successive failures before success is obtained in a Bernoulli trial.
Numerous situations in everyday life utilize geometric distribution. For instance, in the financial sector, a cost-benefit analysis is performed using geometric distribution to determine the financial rewards of a certain course of action.
Looking for last minute help for your AP Statistics exam in May? Find an expert 1-on-1 online AP Statistics tutor from Wiingy and give your exam prep a boost!
What is geometric distribution?
The probability of achieving success for the first time after a series of failures can be expressed as a discrete probability distribution called a geometric distribution. A geometric distribution can attempt an endless number of times up to the first success.
A similar type of distribution is the binomial distribution where there can be only two outcomes i.e., success or failure, and the probability of success is the same for each trial but the difference between Geometric distribution and Binomial distribution is as follows:
Geometric Distribution | Binomial Distribution |
The only success that matters in a geometric distribution is the first one. The random variable, X, keeps track of how many attempts are necessary to get the first success. | The random variable, X, counts the number of successes in the fixed number of trials that make up a binomial distribution. |
Characteristics of geometric distribution
A geometric distribution is defined as a discrete probability of a random variable “x” which satisfies some of the conditions as follows:
- An event that has a series of trials
- And each trial has only two outcomes.
- The probability of success is the same for each trial
Some of the real-life applications of geometric distribution are:
- In sports, namely baseball, a geometric distribution may be used to determine the likelihood that a hitter would receive a hit before receiving three strikes; in this case, the objective is to succeed within three tries.
- In time management, the goal is to complete a task before some set amount of time.
- In cost-benefit analyses, such as a company deciding whether to fund research trials that, if successful, will earn the company some estimated profit, the goal is to reach success before the cost outweighs the potential gain.
The number of Bernoulli trials necessary to achieve the first success is shown by the random variable in the discrete probability distribution known as the geometric distribution.
The outcomes of events that can be counted or are finite are included in a discrete probability distribution. This differs from a continuous distribution, in which results can occur anywhere along a continuum. The binomial, Poisson, and Bernoulli distributions are some examples of discrete distributions.

Properties of geometric distribution
We take the probability of success to be .
And as defined the geometric distribution is a family of curves that models the number of failures before success occurs in a series of independent trials.
- Mean
The predicted value of the geometric distribution would be the distribution’s mean. The weighted average of all values can be used to define the expected value of a random variable, X.
The formula of the mean is
- Variance
The variance is the measure of the dispersion from the mean.
The formula for the variance of geometric distribution is
- Probability mass function
The likelihood that a discrete random variable, , will be identical to some value,
is determined by the probability mass function.
The probability mass function formula is
- Binomial distribution relation
The geometric distribution is a special case of the negative binomial distribution. It deals with the number of trials required for a single success. Thus, the geometric distribution is a negative binomial distribution where the number of successes (
Applications of geometric distribution
Some of the main examples of geometric distribution are:
- Cost-benefit applications
- The majority of organizations often do a cost-benefit analysis using a geometric probability distribution. The goal of a cost-benefit analysis is to calculate the financial gain that an organization would experience from taking a given course of action while deducting the associated costs. This lessens the likelihood of capital loss and enhances the organization’s capacity for decision-making.
- Tossing a coin
- One of the tests that come after the Bernoulli trials is tossing a coin. Let’s say that when a coin is tossed, getting heads on top is deemed a success, and getting tails on top is considered a failure. Geometric distribution makes it simple to depict the likelihood of how many times a coin must be thrown before it lands on its head.
- Other applications:
- Feedback from Customers
- Number of Supporters of a Law
- Number of Faulty Products Manufactured in an Industry
- Number of Bugs in a Code
- A Teacher Examining Test Records
- Playing a Game
- Throwing Darts at a Dartboard
- Number of Network Failures
Identifying the geometric distribution
The three key ways to identify a geometric distribution are:
- There are only two possible outcomes for each trial (success or failure).
- The trials are independent.
- The probability of success is the same for each trial.
If a distribution possesses all the above properties, it can be a geometric distribution.
The geometric distribution is only concerned with the first success in the trials made.
The geometric probability distribution
The geometric distribution is a special case of the negative binomial distribution. It deals with the number of trials required for a single success. Thus, the geometric distribution is a negative binomial distribution where the number of successes (r) is equal to 1.
The formula is:
is the probability of success for each trial
is the probability which is
is the number of failures before the success
is the probability of
successes in
trials.
Standard deviation in geometric distribution
The square root of the variance may be used to establish the standard deviation. The distribution’s divergence from the mean is also shown by the standard deviation.
The following is the formula for a geometric distribution’s standard deviation:
The probability of the geometric random variable
The geometric random variable is used when one is modeling a series of experiments that have one of two possible outcomes – success or failure. The geometric random variable tells you the number of experiments that were performed before obtaining success.
The probability mass function for the geometric random variable is given by:
The variance for this variable is
The cumulative geometric distribution
The chance that a random variable, X, will have a value that is less than or equal to x may be described as the cumulative distribution function of a random variable, X, that is assessed at a point, x. The distribution function is an alternative name for it.
The formula for the geometric distribution CDF is given as follows:
Solved Examples
Example 1: Suppose we are playing a game of soccer. The probability of getting a goal is 0.2. What is the probability we will get a goal on the third try?
Solution:
Given,
Using the formula
Given,
Using the formula and substituting the values we get,
Hence the probability that the person would pick a rotten apple on the 5th try is 0.06561
Example 3: A person trying to sell t-shirts on call observes that 3 out of 63 people who answer want to buy a t-shirt. How many calls does another person who also wants to sell them need to make his first sale?
Solution 3:
As we are looking to calculate the number of calls needed to make only one sale that is the first sale, we need to calculate the expected value of the variable which is also the mean of the distribution.
We have
And
Substituting the value, we get
Hence the number of calls that need to be made to make the first sale would be 12.
Example 4: A die is rolled until a 6 occurs. What is the resulting geometric distribution for the first 3 throws?
Solution 4:
The probability of getting a 6 is
Hence,
Using the formula
Therefore, the geometric distributions for the first three throws are
,
,
Example 5: If the probability of success for a candidate in a voting campaign is 0.5, what is the probability to meet a voter who voted for the winning candidate on your fourth try?
Solution 5:
We have,
And
Substituting the values in the equation
Therefore, the probability is 0.0625.
Conclusion
The geometric distribution is a discrete probability distribution where the random variable indicates the number of Bernoulli trials required to get the first success.
Looking for last minute help for your AP Statistics exam in May? Find an expert 1-on-1 online AP Statistics tutor from Wiingy and give your exam prep a boost!
Frequently asked questions (FAQs)
What is a discrete random variable?
A discrete variable is one that can take on finitely many, or countably infinitely many values.
What does independent trial mean?
Trials in an experiment are independent if the likelihood of each possible outcome does not change from trial to trial.
What is negative binomial distribution?
A negative binomial distribution discusses the ultimate success that may be attained following a string of triumphs in earlier trials. The rth Success in a negative binomial distribution is one that has been preceded by n – 1 trials, each of which contained r – 1 success.
What is the formula of the binomial distribution?
Binomial distribution is a probability distribution used in statistics that summarizes the likelihood that a value will take one of two independent values under a given set of parameters or assumptions.
What is probability?
A probability is a number that reflects the chance or likelihood that a particular event will occur. Probabilities can be expressed as proportions that range from 0 to 1.
References
Gómez-Déniz, E. (2010). Another generalization of the geometric distribution. Test, 19(2), 399-415.
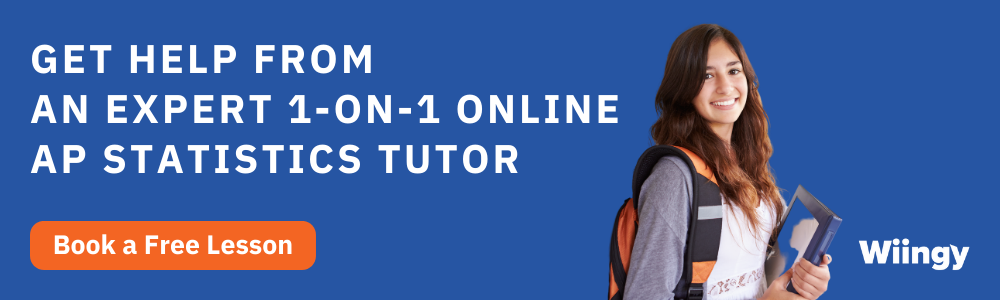
Written by
Prerit Jain