In this article
Types of sampling distribution
Understanding sampling distribution
Estimating the population mean and proportion
Hypothesis testing using sampling distribution
Sampling distributions of the sample proportions
Sampling techniques
Assumptions
Limitations
Solved examples
Conclusion
Frequently asked questions (FAQs)
References
In statistics, the term “sampling distribution” refers to the analysis of several random samples taken from a given population depending on a certain property. The outcomes acquired give a clear picture of changes in the outcomes’ probabilities.
Establishing representative results from small samples of a relatively larger population is its main objective. The population is too big to study; therefore, we choose a smaller group and sample or analyze them again. The obtained information, or statistic, is used to determine the likelihood that an event will occur or probability.
Looking for last minute help for your AP Statistics exam in May? Find an expert 1-on-1 online AP Statistics tutor from Wiingy and give your exam prep a boost!
Types of sampling distribution
- Sampling distribution of mean: It is the probability distribution of each fixed-size sample mean that is chosen at random from a particular population. A graph’s individual means serve to portray a normal distribution. The center of the graph represents the mean of the finite-sample distribution, which is also the mean of that population.
- Sampling distribution of proportion: This kind of finite-sample distribution shows the population proportions. Users choose samples, then figure out the sample proportion. The figures are then plotted on the graph. The average sample proportions obtained from each sample group represent the average sample percentage for the entire population.
- T-Distribution: When the sample size is very small or when the chosen population is unknown to the user, this form of distribution is used. The symmetrical distribution meets the requirements for a typical normal variate. The T distribution tends to approach the normal distribution as the sample size grows. It is used to determine population means, statistical differences, etc.
Understanding sampling distribution
- Normal condition for sampling distribution
- The central limit theorem is used to find the normal condition for sampling distribution. The central limit theorem implies that the distribution of the sample means will be roughly normally distributed if you have a population with mean and standard deviation and take sufficiently enough random samples from the population with replacement.
- Biassed and unbiased point estimates
- Bias is an estimate of how skewed a distribution is. The estimate of the population standard deviation is the standard deviation of the sampling distribution. It is claimed that the standard deviation of the sample distribution is the consistent estimator if it is equal to the standard deviation of the population. So, the smaller the standard deviation from the mean of the sample distribution less biased the population, i.e., if there is an equal spread of the sample around the mean, there is no bias.
- Probability of Sample Proportion
- Sampling is frequently used to calculate the percentage of a population that possesses a particular feature. The population proportion is denoted
and the sample proportion is denoted
.
- Sampling is frequently used to calculate the percentage of a population that possesses a particular feature. The population proportion is denoted
Estimating the population mean and proportion
The central limit theorem is used to calculate the population mean from the sampling distribution.
It can be used when the sample size is larger than thirty and the mean of the sample distribution is the population mean.
The standard deviation of the sample distribution is and the population standard deviation would be
where
is the size of the sample distribution.
Since the value of the sampling and population distribution is the same,
Taking =
.
Hypothesis testing using sampling distribution
All the hypothesis tests use sampling tests to calculate the test statistic. The test statistics have sampling distributions for which the null hypothesis is true.
Instead of taking multiple samples, it is feasible to reliably build sampling distributions using equations. The test statistic is what hypothesis tests perform with sample data.
The analysis inserts the test statistic from the sample inside of its sampling distribution. Hypothesis tests can determine probabilities relating to the chance of getting the sample statistic if the null hypothesis is true since these distributions are a sort of probability distribution. When that likelihood is sufficiently low, the null hypothesis may be disproved.
Some of the tests that use sampling distribution are the chi-square test, z-scores, t-values, and F-values.
Sampling distributions of the sample proportions
The distribution of the sample proportion approximates a normal distribution under the following two constraints:
If both these constraints are followed the sampling distribution of the sample proportion is
- Approximately a normal distribution.
- The sample distribution mean would be equal to the population distribution mean.
- Standard error is equal to standard deviation and is
- If the sampling distribution of
is approximately normal, we can convert a sample proportion to a z-score using the following formula:
This formula is used to find probabilities involving sample proportions.
Sampling techniques
- Simple random sampling
- A subset of a statistical population called a simple random sample is one in which each member has an equal chance of being picked. A straightforward random sample is intended to offer an objective depiction of a group.
- Stratified sampling
- To complete the sampling process, stratified sampling is a form of sampling technique in which the entire population is split into smaller groups or strata. The population data’s common traits serve as the foundation for the stratum. The researcher stratifies the population and then draws a proportionate sample at random.
- Cluster sampling
- A population is divided into clusters and some of these clusters are randomly chosen as your sample via the probability sampling technique known as cluster sampling. In a perfect sample, each cluster would be a tiny reflection of the whole population.
- Multistage sampling
- Multistage sampling is a sampling technique that, to perform research, splits the population into groups (or clusters). Multistage cluster sampling is another name for this advanced type of cluster sampling. Significant clusters of the chosen individuals are divided into sub-groups throughout this sampling technique to facilitate the collection of primary data.
Assumptions
- The sampled values must be independent of each other.
- The sample size,
, must be large enough.
Limitations
- Chances of bias
- The sampling method’s disadvantage is biased selection, which leads us to draw false conclusions. When the method of sample selection is incorrect, bias results.
- Difficulties in selecting truly a representative sample
- Results from challenging sample selection can only be credible and accurate when a sample is entirely representative of the complete population. It might be difficult to choose a sample that is representative of the population when the phenomena being examined are complex. The selection of high-quality samples is difficult.
- Need for subject-specific knowledge
- Utilizing the sampling approach needs sufficient subject-specific sampling procedure expertise. Sampling requires statistical evaluation and estimation of likely error. When a researcher lacks specific sampling knowledge, he may make grave errors. As a result, the study’s findings will be inaccurate.
- The changeability of sampling units
- The sampling method will not be scientific if the population’s units are not homogenous. Even when there are few examples in sampling, it is not always simple to keep with the chosen cases. The sample units might be spread out extensively.
- Impossibility of sampling
- In cases where the universe is either too tiny or too diverse, it is difficult to produce a representative sample. Census research is the only alternative choice in this circumstance. Additionally, the sampling method might not be suitable for studies that need a high degree of precision. The most meticulous sample selection still leaves room for error.
Solved examples
Example 1: Given that 45% of Americans own a Dell laptop. Taking a random sample of 50 Americans observed, calculate the probability the proportion of the sample who own a dell laptop is between 47% and 50%.
Solution 1:
Its given that is 0.45 and
is 50.
We need to check the constraints for the sampling of the distribution
and
both are greater than 15 hence the constraints satisfied.
Hence the sampling distribution will have mean as a normal distribution which is equal to .
Standard deviation will be calculated using the formula.
Now we need to find the probability of it lying between 47 and 50.
Therefore, there would be a 14.98% chance that we would see a sample proportion between 47% and 50% when the sample size is 50.
Example 2: The number of people in a household has a mean of 2.5 and the standard deviation is 1.7. What is the probability that the mean size of a random sample of 81 households is more than 2?
Solution 2:
Using the central limit theorem we can say the mean of the sample is the same as the mean of the population which is 2.5
Now to find the standard deviation we use: .
Which
Now we need to find the probability that it is greater than 2 i.e.,.
This implies that there is a probability that the households have more than 2 people is 0.0028 which implies that the sample is skewed.
Example 3: The mean and standard deviation of a certain population are and
. Suppose random samples of size 100 are drawn from the population. What are the mean
and standard deviation
of the sample mean
?
Solution 3:
Since we can say that
=
.
And
Hence the meanis 15 and the standard deviation of the sample mean is 0.41
Example 4: It is given that 30% of Americans use a google smartwatch. If a random sample of 50 Americans were taken, calculate the probability the proportion of the sample who use a google smartwatch is between 35% and 40%.
Solution 4:
It’s given that is 0.30 and
is 50.
We need to check the constraints for the sampling of the distribution and
, are both greater than or equal to 15 hence the constraints satisfy.
Hence the sampling distribution will have mean as a normal distribution which is equal to
Standard deviation will be calculated using the formula .
Now we need to find the probability of it lying between 35 and 40.
Therefore, there would be a 15.39% chance that we would see a sample proportion between 35% and 40% when the sample size is 50.
Example 5: The numerical population of grade point averages at a college has mean 2.5 and standard deviation 0.50. If a random sample of size 100 is taken from the population, what is the probability that the sample mean will be between 2.4 and 2.8?
Solution 5:
For the sample mean
It’s given that is 2.5 and
is 100.
Standard deviation will be calculated using the formula:
Now we need to find the probability of the sample mean lying between 2.4 and 2.8.
Conclusion
Sampling distributions are useful tools used by researchers to estimate and draw conclusions about a wider population of interest. We can use these sample distribution data visualizations and can draw accurate conclusions and gain a better knowledge of a group as a whole.
Looking for last minute help for your AP Statistics exam in May? Find an expert 1-on-1 online AP Statistics tutor from Wiingy and give your exam prep a boost!
Frequently asked questions (FAQs)
Why is sampling distribution important?
It is crucial to gain a graphical depiction to comprehend how much the result of an event could change. Additionally, it aids users in comprehending the demographic they are interacting with.
What is the proportion?
A proportion is a part, share, or number considered in comparative relation to a whole. It can be equal to 0, 1, or any value between 0 and 1. It can be expressed as a number or percentage.
What is the definition of probability?
Probability is a measure of the likelihood of an event occurring.
What is the confidence interval?
A confidence interval refers to the probability that a population parameter will fall between a set of values for a certain proportion of times.
What is a normal distribution?
A probability distribution that is symmetric around the mean is the normal distribution, sometimes referred to as the Gaussian distribution. It demonstrates that data that are close to the mean occur more frequently than data that are far from the mean.
References
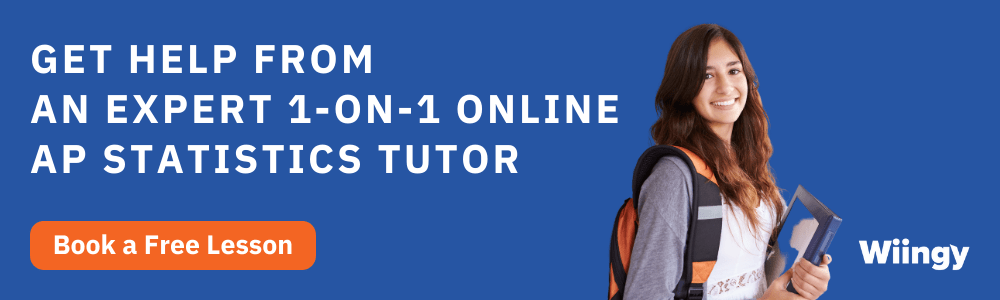
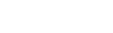
Mar 25, 2025
Was this helpful?