What is data collection?
Data collection in Statistics refers to the process of compiling information from all pertinent sources in order to resolve the study topic. Evaluating the result of the issue is helpful. One might get to a conclusion about the answer to the pertinent issue using data-collecting techniques.
The majority of firms employ data-collecting methods to predict probability and trends in the future. After the data has been gathered, the process of organizing the data must be done.
Data will be gathered in order to study and make judgments on a certain business, sales, etc. The information gathered will be used to draw some inferences about how well a certain company is performing.
As a result, data collecting is crucial for problem-solving, establishing assumptions about certain things, and analyzing the success of a business unit. We will be examining what data gathering is and how it benefits different areas before moving on to the techniques of data collection.
Looking for last minute help for your AP Statistics exam in May? Find an expert 1-on-1 online AP Statistics tutor from Wiingy and give your exam prep a boost!
Planning a study
Sample planning is a thorough breakdown of the measurements to be made:
- Time: Choose the appropriate time to conduct the survey. For instance, gathering opinions from the community before a new article in the region launches.
- Category: Choose the sample techniques that will be used to choose the subjects for the survey.
- Material: Make a decision about the subject matter for the survey. It could be a paper checklist or an internet survey.
Steps in sample planning
- Parameter identification: Identify the qualities or characteristics to be measured. Determine the possible values, ranges, and needed resolution.
- Decide on a sampling strategy that includes specifics like how and when samples are to be selected.
- Choose Sample Size: Choose a sufficient sample size to accurately reflect the population. Large samples are typically more likely to result in false conclusions.
- Storage: Choose a data storage format in which the sampled data will be saved by selecting a storage type.
- Assign Roles: Assign roles and duties to each individual participating in the phases of data collection, processing, and statistical testing.
- Verify and carry out: A sampling strategy should be able to be verified. Send it to associated parties for execution when it has been validated.
Identifying a sample and population
The whole group about whom we wish to make conclusions is referred to as a population.
The particular group from which you will gather data is known as a sample. The sample size is always less than the population as a whole.
- Why is sample and population in a study important?
Because it is typically impractical to investigate the complete population, studies are done on samples. Conclusions made from samples are meant to be extrapolated to the entire population, and occasionally even to the future. Consequently, the sample must be representative of the population. The easiest way to do this is to employ appropriate sample techniques. In fact, neither more nor less must be used; the sample must be of an appropriate size.
- Generalizability of survey results and its importance
The generalizability of a study refers to how well its findings may be used in a wider context. When the findings are generally applicable to most situations, most individuals, and most of the time, this is referred to as generalizability.
Generalizability is important because:
- The randomness of the sample, with an equal probability of selection for each study unit.
- How accurately the sample represents your population?
- The sample size, with bigger samples, has a higher likelihood of producing statistically significant findings.
Sampling methods
There are two main types of sampling methods.
1. Probability sampling
The probability sampling technique makes use of a random selection technique. In this strategy, every eligible person has a chance to choose a sample from the whole sample space. This approach takes longer and costs more money than the non-probability sampling approach. The advantage of probability sampling is that it ensures the sample will accurately reflect the population.
Types of probability sampling
- Simple random: Every item in the population has an equal and likely probability of being chosen for the sample when using a basic random sampling procedure. This approach is referred to as the “Method of Luck Selection” since the decision to pick an item is solely based on chance. It is referred to as “Representative Sampling” since the sample size is substantial and the item was selected at random.
- Systematic: By choosing the random selection point and then choosing the other methods after a predetermined sample interval, the items are chosen from the target population in the systematic sampling approach. By dividing the entire population by the required population, it is computed.
- Stratified: To finish the sampling procedure, the entire population is separated into smaller groups using a stratified sampling approach. The tiny group is made up of people who share a few traits with the general population. The statisticians choose the sample at random after dividing the population into smaller groups.
- Clustered: The population set is used to create the cluster or group of individuals in the clustered sampling technique. Similar significant traits apply to the group. Additionally, they have a comparable likelihood of being included in the sample. Simple random sampling is used in this approach to sampling the population cluster.
2. Non-probability sampling
In contrast to random selection, we choose the sample in the non-probability sampling approach based on their own assessment. With this methodology, not every person in the population has the opportunity to take part in the research.
Types of non-probability sampling:
- Convenience: In a convenience sampling strategy, the samples are chosen directly from the population since we can easily access them. The samples are simple to choose, and we can avoid selecting the sample that best represents the population as a whole.
- Consecutive: With a small difference, consecutive sampling is comparable to convenience sampling. A single individual or a group of persons is chosen by us for sampling. We then conduct a further study for some time, analyze the findings, and, if necessary, switch to a different group.
- Quota: The quota sampling approach includes creating a sample of people to reflect the population based on certain characteristics or attributes. We select sample subsets that produce an informative data set that generalizes to the full population.
- Purposive: In purposive sampling, just our knowledge is used to choose the samples. As our expertise is used to create the samples, there is a probability of receiving extremely accurate responses with little tolerance for mistakes. It is often referred to as authoritative sampling or judgmental sampling.
- Snowball: Chain-referral sampling is another name for the snowball sampling technique. The samples in this approach contain characteristics that are challenging to identify. So, each element of the population that has been identified is requested to locate the other sample units. These sample units are a part of the same intended audience.
Sources of bias in sampling methods
When certain individuals of a population are consistently more likely to be chosen in a sample than others, this is known as sampling bias. In the medical sciences, it is also known as ascertainment bias.
Because sampling bias jeopardizes external validity, particularly population validity, it restricts the generalizability of findings. In other words, results from skewed samples can only be extrapolated to populations with similar traits.
- Causes of bias:
- Sampling bias in probability samples: Every member of the population has a known chance of getting chosen in probability sampling. For instance, you may choose a straightforward random sample from your population using a random number generator. Although this method lowers the chance of sampling bias, it could not completely remove it. A biased sample might be produced if your sampling frame—the actual list of people from whom the sample is drawn—does not correspond to the population.
- Sampling bias in non-probability samples: The selection of a non-probability sample is made using non-random criteria. For instance, individuals in a convenience sample are chosen based on their accessibility and availability. Non-probability sampling frequently yields skewed samples because certain population members have a higher likelihood of inclusion than others.
- How to avoid bias in sampling methods: You may prevent sample bias by carefully planning your study design and sampling techniques.
- Define a sampling frame and a target population (the list of individuals that the sample will be drawn from). To lessen the chance of sampling bias, try to match the sample frame as closely as possible to the target population.
- Make online surveys as brief and user-friendly as you can.
- After non-responders, follow up.
- Steer clear of convenience sampling.
- When members of specific groups are underrepresented, sampling bias can be avoided by using oversampling. This is a technique for choosing responders from certain categories such that they represent a bigger percentage of a sample than they do of the population as a whole.
To eliminate any sampling bias, answers from oversampled groupings are weighted according to their actual proportion of the population after all data has been gathered.
Designing an experiment
A set of techniques is developed through experimental design to systematically examine a hypothesis. A thorough grasp of the system you are researching is necessary for a successful experimental design.
Steps to design an experiment:
- Think about your variables and their relationships:
- Start by formulating a clear research question. We’ll practice with two examples of research questions from the fields of ecology and health sciences.
- Create a precise, verifiable hypothesis:
- We ought now to be able to formulate a precise, testable hypothesis that responds to your research question now that we have a solid conceptual grasp of the system you are researching.
- Create test procedures to alter your independent variable:
- We must find the degree to which the results may be extended and used in a larger environment can be influenced by how the independent variable is controlled in the experiment. We may need to choose your independent variable’s range of variation first.
- We might also need to decide how precisely to alter your independent variable. Our experimental system may make this decision for you occasionally, but more often than not, we will have to make our own option, which will impact how much we can deduct from our data.
- Subjects should be divided into groupings, either within or between subjects:
- We must first think about the study’s sample size or the number of participants. The statistical power of our experiment, which affects how much confidence we may have in our results, is often increased when we have more people.
- Prepare a plan for measuring your dependent variable:
- The final step is to choose the methodology for gathering data on the results of our dependent variable. We should strive for accurate measurements with little bias or inaccuracy in the research.
- Science-based tools can be used to measure some variables objectively, such as temperature. To make them measurable observations, some may need to be operationalized.
Random sampling vs. random assignment
We can get a sample that is typical of the population by using random sampling.
As an outcome, the public can use the study’s findings.
We can ensure that the sole distinction between the multiple treatment groups is the subject of our study thanks to random assignment.
As a result, causality might be assumed.
Conclusion
In this article we learned about collecting data and why the right way to do it is so important for statistical analysis. We learned how to identify a sample and a population and the different types of sampling. We also saw what sampling bias is and how it is caused and how it can be avoided. We learned the steps to design an experiment and what random sampling and assignment are. The collection of data is a very vital procedure for analyzing a lot of day-to-day activities.
Solved examples
Example 1: In the research of time using a phone before sleep find the independent variable and dependent variable.
Solution 1:
The independent variable would be minutes of phone use.
The dependent variable would be hours of sleep per night.
Example 2: In the same research as above find the extraneous variable and how to control it.
Solution 2:
The extraneous variable would be individual differences in sleep patterns that are caused by nature.
Measure the average difference between sleep when using a phone and sleep while not using a phone as a statistical control instead of the average quantity of sleep for each treatment group.
Example 3: Write a null hypothesis for the example one experiment.
Solution 3:
: The quantity of sleep a person receives does not connect with using a phone before bed.
: A decline in sleep is caused by increasing phone use before bed.
Example 4: For the Example 1 case put the participants in different treatment groups (completely randomized and randomized block).
Solution 4:
Completely randomized: Utilizing a random number generator, a level of phone use will be allocated to each subject at random.
Randomized block: Prior to assigning phone use treatments within these categories, subjects are initially classified by age.
Example 5: For Example 1 research find the within the subjects and between the design of the subject.
Solution 5:
Within the subjects: Through the course of the trial, subjects are randomized to receive zero, low, or high degrees of phone use in the following order.
Between the subjects: Randomly chosen levels of phone use—none, low, or high—are given to subjects, and they stick to those levels for the duration of the trial.
Looking for last minute help for your AP Statistics exam in May? Find an expert 1-on-1 online AP Statistics tutor from Wiingy and give your exam prep a boost!
Frequently asked questions (FAQs)
What is a confounding variable?
An additional variable in a study looking at a potential cause-and-effect link is known as a confounding variable, also known as a confounder or confounding factor.
What is internal validity?
The level of assurance that the causal link you are examining is not impacted by other variables or circumstances is known as internal validity.
What is external validity?
The degree to which your findings may be extrapolated to different situations is known as external validity. The experiment’s validity will rely on how it was designed.
What is the statistical hypothesis?
A description of a population’s makeup. It is frequently expressed as a parameter of the population.
What is sampling error?
The discrepancy between a population parameter and a sample statistic is known as a sampling error.
References
Pham, H. (Ed.). (2006). Springer handbook of engineering statistics (Vol. 49). London: Springer.
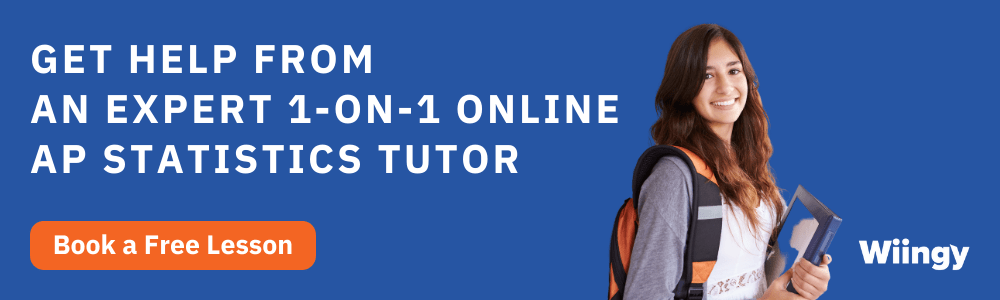
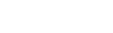
Mar 10, 2025
Was this helpful?